Friday, June 01, 2012
Today Kenneth Hamilton and I presented at the Society for Scholarly Publishing
(SSP) 2012 conference. Below are the slides, which are also available on the
Helioid
blog. Additionally, here is a brief post on the SSP
Startup Panel and our co-presenters.
Monday, April 02, 2012
Today I will be presenting a poster at ECIR 2012 about a paper Katja Hofmann
and I have written. The abstract and full paper are included below.
The abstract:
In recall-oriented search tasks retrieval systems are privy to a
greater amount of user feedback. In this paper we present a novel method of
combining relevance feedback with learning to rank. Our experiments use data
from the 2010 TREC Legal track to demonstrate that learning to rank can tune
relevance feedback to improve result rankings for specific queries, even with
limited amounts of user feedback.
P. Lubell-Doughtie and K. Hofmann, "Learning to Rank from Relevance Feedback
for e-Discovery," in ECIR, 2012.
Monday, January 16, 2012
Simple math and CSS are
used to create the below heat map showing the results of the All Our Ideas Wikipedia
Banner Challenge.
Pairwise data collection is particularly suited to matrix based
visualization. We use intuitive colors so that after quickly skimming the
results your gaze naturally drifts towards the better and worse banner
pairs.
Monday, August 29, 2011
This presentation concerns a method I developed to use user interactions
with search results to automatically re-rank results and thereby produce a
higher-quality ranking, which increases in quality with more user interaction.
Below is a presentation showing the method and results:
Download the above presentation
and the complete thesis.
Friday, August 26, 2011
I will be defending my thesis, Learning to Rank from Relevance
Feedback, Monday August 29th at 1500 in room G.005 at Science
Park.
Below is a schematic of the method I developed to learn and re-rank documents
as users browse through them:
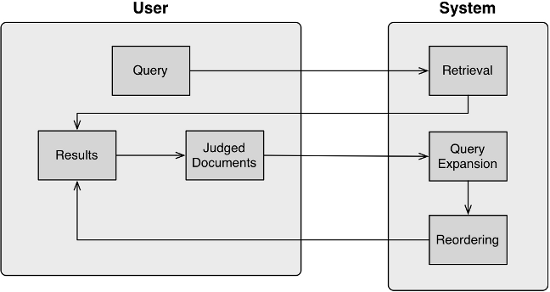
The abstract of my thesis is below. I will post the full text next
week.
When searches involve ambiguous terms, require the retrieval of many documents, or are conducted in multiple interactions with the search system, user feedback is especially useful for improving search results. To address these common scenarios we design a search system that uses novel methods to learn from the user's relevance judgements of documents returned for their search. By combining the traditional method of query expansion with learning to rank, our search system uses the interactive nature of search to improve result ordering, even when there are only a small number of judged documents. We present experimental results indicating that our learning to rank method improves result ordering beyond that achievable when using solely query expansion.