Sunday, June 05, 2011
The Helioid team and collaborators have released a Europe
Travel Guide for Android.
We have packed over
3600 European destinations in a tiny offline guide. It is filled with
great content from wikitravel and is the perfect guide for
backpackers.
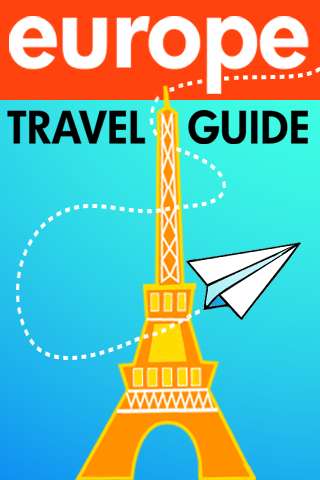
- Up-to-date info about transport and accommodation options
- Hundreds of helpful phone numbers, email addresses, and URLs
- Dining and shopping guides for major cities
- Country-specific safety and emergency contact information
- Extensive information about local culture and customs
Available on on
Android Market now.
Monday, May 16, 2011
Below are the slides from a presentation I gave
at the Natural Language Processing & Learning Workshop.
I presented my project for the Unsupervised Language Learning class,
entitled "Unsupervised Constituency Grammar Induction: Learning Bracketing and
Phrasal Categories."
Thursday, February 24, 2011
A couple months ago we opened up the Helioid development version to the
public, but have not yet announced it. We have recently migrated servers and
tweaked algorithms and have significantly improved the performance. There is still a lot of work to be done but we believe this reflects a healthy starting point.
The graphic below illustrates how you can use Helioid to go from a question or idea to a
organized lists of results that helps you build better search queries.
This version of Helioid is a meta-search engine offering category based personalization. Below is
screenshot of the results after querying Helioid:
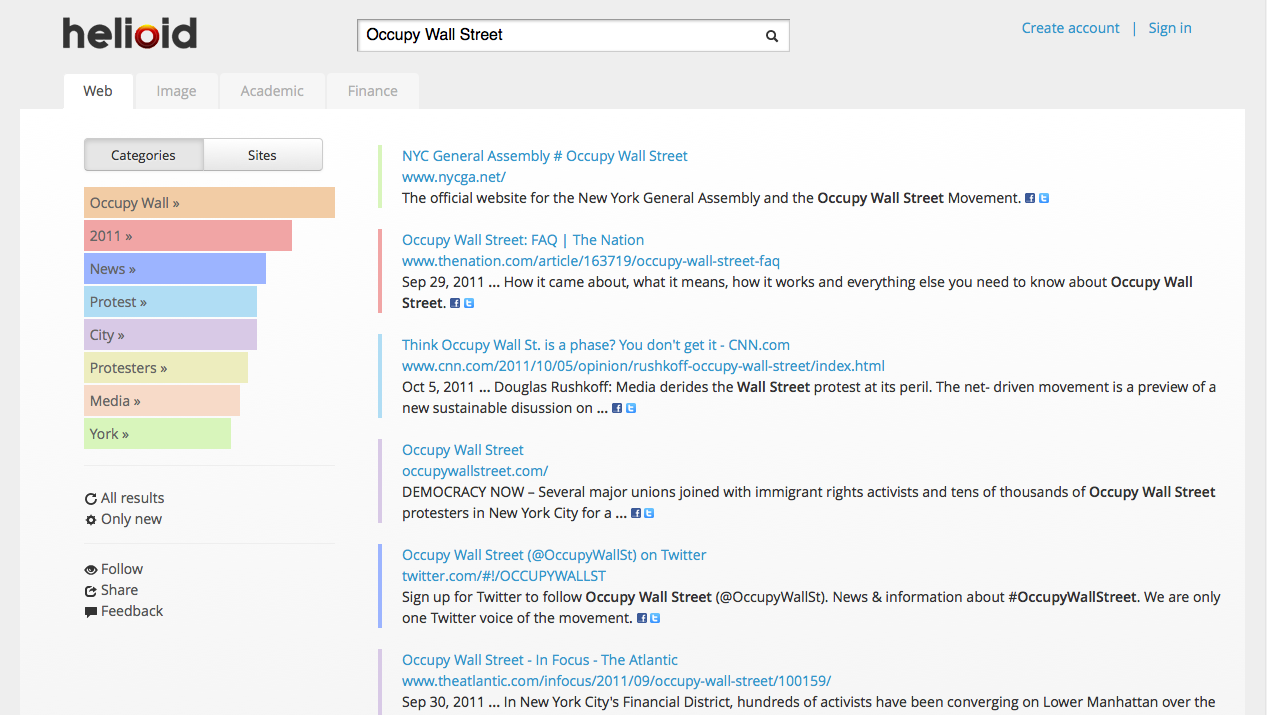
By interacting with the categories on the left you can choose the results to
be shown on the right. Please let us know if you have any suggestions,
comments, criticisms, complaints; anything. Try out the Helioid preview now.
Thanks to Ben de Jesus for helping
with site design and user experience.
Monday, February 07, 2011
Katja and I presented a poster regarding our paper entitled "Improving Result
Diversity using PLSA" at DIR 2011 on Friday. The poster is below:
The abstract is included below. The full
paper is available for download as well.
IA-SELECT is a recently developed algorithm for increasing the
diversity of a search result set by reordering an original document list
based on manually generated clusters. In this paper we extend this approach
to create a diversification framework in which arbitrary clustering methods
can be used, and where the influence of clusters can be balanced against
the original rank of documents. We study whether clusters that are
automatically generated using probabilistic latent semantic analysis (PLSA)
can compete with manually created clusters, and investigate how balancing
the influence of clusters and original document rank affects diversity
scores. As there are currently few datasets for evaluating diversity, we
develop a new dataset, which is released with this paper. Our results show
that diversification using PLSA can improve diversity, but that there is a
large gap in performance between automatically and manually created
clusters.
Monday, November 09, 2009
Update 02/2011:
I just became aware of The Serval
Project which addresses the same idea:
Communicate anywhere, any time … without infrastructure, without mobile towers, without satellites, without wifi hotspots, and without carriers. Use existing off-the-shelf mobile cell phone handsets. Use your existing mobile phone number wherever you go, and never pay roaming charges again.
They have code for their
phone app and their
distributed naming system available.
Below I present the idea for a P2P Telecom. That is, a telecom in which traffic is routed from sender to receiver through peers. Not through a central hub controlled by a company.
Cons against this implementation.
- You will only benefit from a P2P telecom if the people you're communicating with are using the P2P telecom.
Pros in favor of a P2P Telecom.
- Since users will be controlling the network, users will be setting the prices and it will benefit all users for prices to be as low as possible. This is a stark contrast to the current system where prices are set by a handful of powerful companies with little worry of losing subscribers.
- No additional infrastructure will need to be put in place. In fact, towers, antennas, relays, etc. can be removed from densely populated areas because they are unnecessary for a P2P Telecom to function.
- A P2P Telecom will have more privacy because you will trust no one but yourself and encryption levels you control. If you want to use a theoretically unbreakable but data intensive encryption protocol that's up to you and no one will know your data but you and the other user you are communicating with. Like any communications system, the privacy of a P2P Telecom is not perfect but, as any cryptographer worthy of their title will tell you, the system is open and this is leaps and bounds more secure than the closed systems of big industry telecoms.
- There is no centralized control. This helps to enable better data security and increased privacy, among other things. Without centralized control no one will be able to shut off the network and there will be no data overloads in case of an emergency (such as happened in New York City during September 11, 2001).
- There will be increased capacity. This lack of centralized control enables increased capacity and redundancy in populated areas beyond anything approachable by current industry telecoms.
- A P2P Telecom can transfer any form of data. Because of their popularity phone calls are the most obvious forms of data to be transferred but if desired the network can transfer anything else such as video or raw data.
It is clear to me that the pros of a P2P Telecom definitively outweigh the
cons and it is time we start building this network, which turns out to be
rather simple.
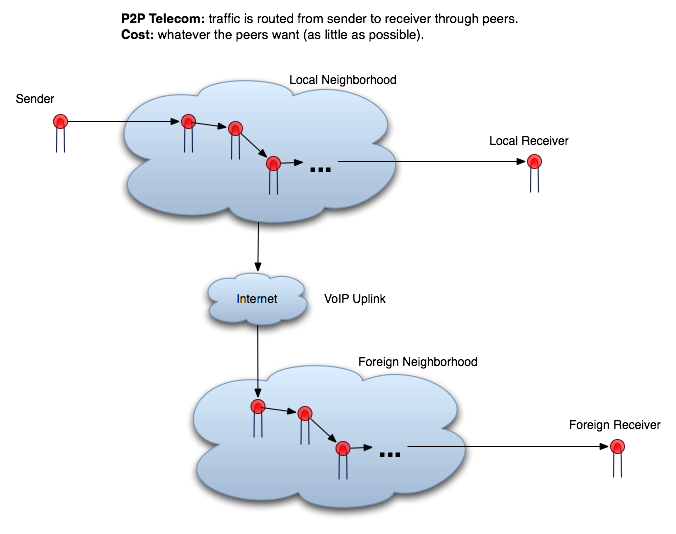
The Implementation of a P2P Telecom
The implementation is based on modifying the functionality of existing cell
phones be they GSM, CDMA, or some other unheard of system.
Cell phones must be retrofit so they can act as peers with one another. At
their core cell phones send and receive signals, these phones must be modified
so that they can receive and send signals directly from and to other
phones.
- Accomplishing this may or may not require hardware modification. If hardware modification is required we must determine how this can be done. One simple method would be the use of a modified SIM chip. If the change needed is more fundamental a systematic approach to performing it can be developed.
- Software modification will be required. We must:
- Change what type of signals the phone looks for so that it can find signals from other phones
- Change how the phone interprets the signals it finds so that it can interpret them as:
- Data passing. The phone must know how to move data from itself to other peers. This will require an algorithm informing the phone of the priorities for where to forward data it receives. This algorithm will benefit from, but not require, partial location awareness of peers and unique identification of peers. This can all be done while maintaining anonymity.
- Data receiving. The phone must know that this data is destined for it and know how to handle decoding of the data.
- Data sending. The phone must be able to uniquely encoded it's data so it is only decodable by the receiver.
The Long Distance Uplinks in a P2P Telecom
A problem with the described P2P telecom is that if you want to call
someone outside of your peer neighborhoods range or someone who doesn't use
the P2P system you'll have to place the call over the existing industry
telecom network. A solution to this problem is to give every P2P neighborhood
an internet uplink and connect multiple neighborhoods with a VoIP services.
In this manner, if either of the above situations occur, the call can be
placed using VoIP technologies.
The number of peers with access to the uplink and therefore the bandwidth
of the circuit between the peers and the uplink will depend on the volume of
calls going out of the local neighborhood.
The Algorithms for a P2P Telecom
- Finding a peer and establishing a circuit
- Sending data through a circuit - Encrypt data using public/private key system
- Throttling of uplink bandwidth - Better to deny calls that increase latency on calls
- Location anonymnity