Sunday, March 15, 2020
COVID-19 Time Series Analysis
Here we analyze the COVID-19 case data to create simple charts and time series models. This is meant as a review of time series analysis methods and not to accurately predict the future course of the pandemic. These models are based on the daily case data only, they are all univariate, and they do not consider environmental changes (e.g. travel restrictions, quarantines, and event cancellations).
The rest of this post describes the functionality of the COVID-19 Time Series Analysis IPython Notebook and includes charts with the data available as of March 15th, 2020. Feel free to use, modify, extend, and contribute to the notebook.
Fetch COVID historical case date
Each time we run the build_data
function we will:
This fetches all data available in the CSSEGIS COVID-19 repository at the time it is run.
Use the unify_names
function to combine multiple countries and/or provinces/states into a single country. This resolves problems when the names of single countries are inconsistent in the CSSEGIS datasets.
Use the plot
function to produce total cases and case per day charts for a set of countries
and metrics
, passed as parameters.
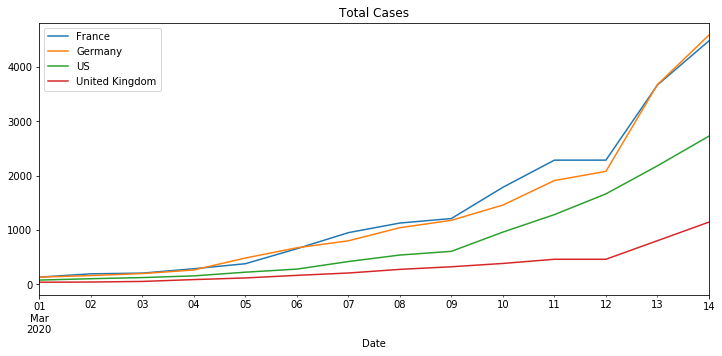
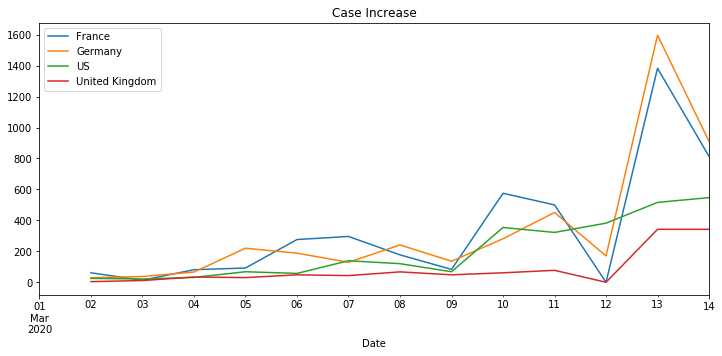
Naive Time Series Models
This section requires that the grouped_df
DataFrame and the all_countries
list exist. These models are not run in parallel, but they can be. These models are incredibly naive, we are only considering the previous case numbers, nothing exogenous.
Use the prepare_data
function to limit the countries and metrics. Currently this can model univariate data, i.e. it only considers a single country (or province/state) at a time. It would be interesting to use VAR
or other multivariate models to capture the interdependence between countries.
We have written forecasting functions that use the ARIMA and Holt Winters Exponential Smoothing time series analysis methods. Additional forecasting methods in the same format as these functions should work fine.
The grid_search
function takes a map from forecasting functions to a list of configurations per forecasting function and evaluates all configurations using a walk forward method with a 2/3rds training and testing split. There is no validation or cross-validation step. This function returns a list of forecast function, configuration, and mean squared error (MSE) tuples ordered by increasing (i.e. worse) MSE.
On March 15th, 2020, when evaluating the US case count, an ARIMA model with order (2, 1, 4) had the lowest MSE of 4,933. The below chart plots the actual and predicted values for the testing data.
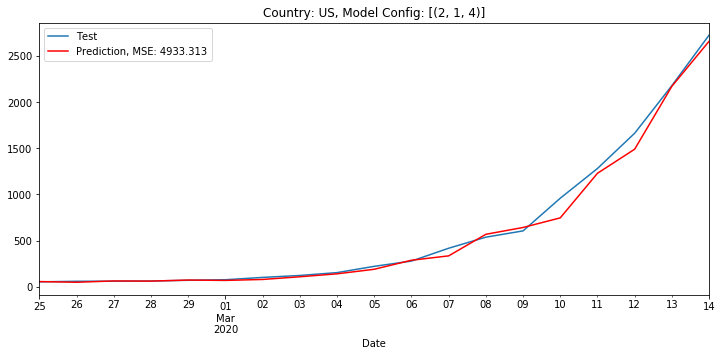
Below is the result if we have this best fit model (best as in lowest MSE) predict the number of cases for the next two weeks. The gap is because we do not connect the last day of actual data to the first day of predicted data. We show predicted cases as a red dotted line.
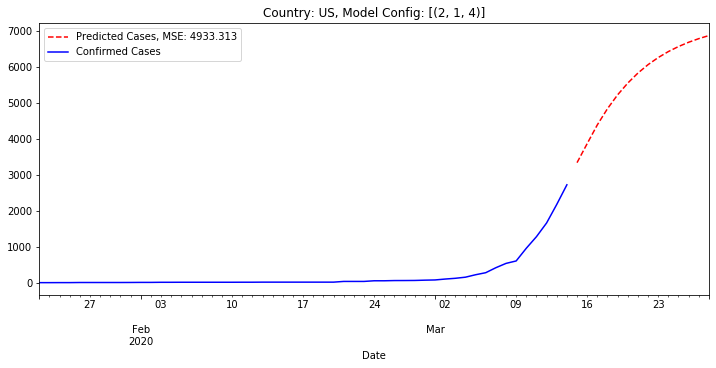
Looking at a wider set of models, below is the result for the next week showing all models with a MSE below 20,000 in pink and the best model in dark red. We see that when considering all our models there are a variety of possible outcomes.
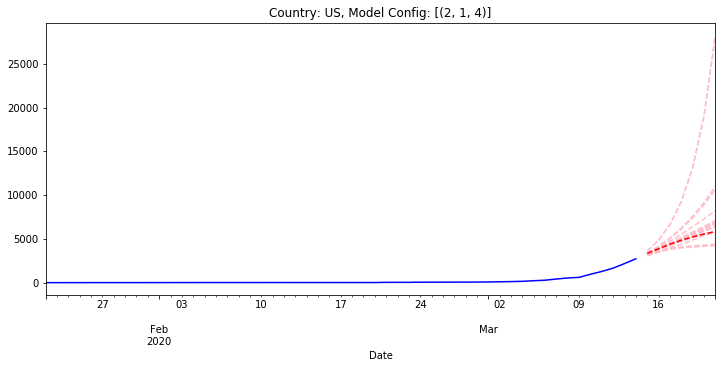
There are many other factors and methods that would improve these models. This is meant as a review of time series analysis methods and not to accurately predict the future course of the pandemic. Please take a look at the COVID-19 Time Series Analysis IPython Notebook to explore and extend the code.
Wednesday, February 28, 2018
An essential part of ensuring that people have equitable access to services is being able to quickly and continuously assess whether those services are functioning properly. If your government provides health care it needs to know those clinics are open, if it provides public bus services it needs to know those buses are running on time, and if it provides water access it needs to know that water is flowing.
By training a deep learning model we are able to predict, from an image, whether a water point is functional or not with around 80% accuracy. Analyzing network activations shows that the model appears to be identifying a latent structure in the images. Given this, we expect the prediction accuracy to increase as the dataset expands.
Introduction
We’re involved in ongoing research to build better (e.g. cheaper, faster, more accurate) ways to answers these service delivery questions on behalf of our partners. Towards that end we recently ran some experiments to see if we can use labeled datasets to predict, from only an image of a water point, whether that water point was functioning or not. This lets us create models which, given an input image, output a prediction of whether that water point is working or not working. A model like this can help governments, civic organizations, and other actors in the development sector in at least two ways:
- An enumerator can take a picture of a water point and see if the model predicts that it is working or not, thereby validating their work.
- We can run the model against a set of historical image data to see where the model disagrees with the recorded values, and use this to flag potentially inaccurate data.
Image classification using neural networks has a long history going back to Yann LeCun’s work using convolution neural networks for digit recognition with LeNet. Our specific task of boolean (yes or no) classification for generic images has also attracted a lot of attention, such as with Kaggle’s Dogs vs Cats challenge. There’s a more generic annual challenge to build models from labeled ImageNet data to classify image content as well as multiple objects within an image. More recently, Google’s AutoML now provides a higher-level UI for building an image classifier.
Learning Method
To assess whether the general problem of predicting categorical column values from images in our domain is feasible, we use neural networks to predict whether a water point is functional or not from an image of the water point. We performed this experiment on the water point dataset because we expect there to be a relationship between the water point images and their functional status, and because this model can provide utility to our partners. We started with the basic model described by Francois Chollet in Building powerful image classification models using very little data. We then extended this model by adding an additional convolutional layer, increasing the range of image transformations, and varying the type and parameters of the model optimizer.
To generate the inputs to the model we first split the dataset by type of water point and then built separate models for each type. The original images are 1,836 by 3,264 pixels in both portrait and landscape format. To prepare them for training we used the EXIF information to rotate them as needed and reduced the resolution to 150 by 150 pixels. Additionally, we used Keras’ image generator to add rotated, sheared, zoomed, width and height shifted, and horizontally flipped versions of the images. Considering only hand pump type water point images, we have 1,605 examples, which we divide into 400 validation images and 1,205 training images.
The image above shows a diagram of the network we used for prediction. It is a feed forward neural network with four 2D convolutional layers of 32, 32, 64, and 128 nodes, all using a rectified linear unit activation function and a 2 by 2 pool size. This is followed by a fully connected layer of 128 nodes and a dropout layer to prevent overfitting. Finally, we use a sigmoid activation function to model the single class binary output of functioning or not-functioning.
We experimented with a number of different dropout rates, optimizers, learning rates, and decay rates. We tested the optimizers Adam, AMSGrad, Adagrad, Adelta, Adamax, Nadam, and RMSProp using a coarse grid search over the learning rate and dropout proportion hyper-parameters [1, 3]. We evaluated learning rates from 10-3 to 10-5 and dropout proportions from 0.4 to 0.7.
Results
We found that a learning rate of 10-4 provided the best results. Nadam did not produce good results, but the other optimizers performed reasonably well, all leveling off after around 100 training epochs. A dropout proportion of 0.5 appeared to perform the best, with performance increasingly negatively affected farther from that value.
The above graph shows the loss and accuracy for the training and validation data over 100 epochs. With accuracy higher is better, whereas with loss lower is better. We see that over time both the training and validation set accuracies increase, while the losses decrease. This particular model was trained using the Adam optimizer with a learning rate of 10-4, dropout of 0.5, and batch size of 32. After 100 epochs the validation accuracy appears to have stabilized.
At the 100th epoch the training loss is 0.3572, training accuracy is 0.8433, validation loss is 0.4572, and validation accuracy is 0.7772. The validation accuracy measures how the model performs when evaluated on a set of images that it has never seen before. This is a useful proxy for how we would expect it to perform in the real world. We can therefore expect this model to correctly predict the functional status of around 80% of handpump waterpoint images.
To gain insight into how the model is creating its predictions we will look at a couple of example classifications. The left hand column below shows scaled waterpoint images, and the right hand column shows the activation values for that image from a section of the neural network.
The model correctly predicts that the top image above is a functional water point and that the bottom image is not functional. In this model each image is given a score between 0 and 1, with 0 meaning the model is confident the waterpoint is functional, and 1 meaning the model is confident the waterpoint is broken. The top image received a score of 0.00774 and the bottom received a score of 0.907150.
The network activation images in the right-hand column above indicate greater activation through lighter colored pixels and vice versa. Network activation images are challenging to interpret, but the model seems to be using the existence of brown, muted colors as indicative of functional status. You’ll notice that in the top row, the brighter grass and blue bucket colors in the image appear inactive in the neural network. Alternatively, the large patch of beige parched land at the top of the bottom image leads to high network activation, as shown by the bright points at the top of that image’s activation graph.
Conclusion and Future Work
Along with increasing the number of samples to improve accuracy, there are potentially fruitful avenues to improve accuracy by increasing the amount of data in each sample. We can do this by adding existing organic data to the learning algorithm, e.g. location and time the image was taken, and by adding synthetic data, e.g. the population density at that location and time. Of course when expanding the input data it will be important to take into consideration the use case for the model and distinguish between what we can expect to know from what we are trying to predict.
There are also a number of improvements to the model that we can experiment with. The current hyper-parameters were chosen with a coarse grid search, we should do a fine grid search over the hyper-parameters and add additional hyper-parameters to the search space, including pool size and optimizer. We should experiment with additional network architectures, both deeper and wider. Given the complexity of the problem there’s good reason to believe our network cannot express the topological complexity of the dataset [2]. Although preliminary tests did not produce good results, we should also experiment further with transfer learning using pre-existing network weights, such as ImageNet.
This is only the start of an ongoing project and there are an exciting number of directions for future work. With these building blocks, we’re moving towards a toolset where creating a predictive model from your dataset is as easy as creating a chart.
A Python notebook for the model we used above is available in the dl4d repository. The image transformation code is a heavily modified version of Carin Meier’s Cats and Dogs With Cortex Redux.
Cross posted from Ona’s blog.
Further Reading and References
- Duchi, Hazan, and Singer, Adaptive Subgradient Methods for Online Learning and Stochastic Optimization
- Guss and Salakhutdinov, On Characterizing the Capacity of Neural Networks using Algebraic Topology
- Kingma and Ba, Adam: A Method for Stochastic Optimization
Thursday, August 31, 2017
A common need across all our projects and in our partners projects is to build up-to-date indicators from stored data. In our malaria spraying project (mSpray), our work monitoring the drought response in Somalia, and our electronic medical record system work for OpenSRP, we need to build dashboards showing the progress of the project and other information relevant to stakeholders. Currently we do this on an ad-hoc basis, but we are in the process of building a unified pipeline to move data from heterogenous systems into a data warehouse and build indicators on top of this data.
This need breaks down into the following minimal requirements:
- Store data
- Store queries relative to the data
- Retrieve the results of queries executed against the latest data
The queries may be complex, e.g. containing grouping, filtering, and aggregation clauses, and the datasets may be large, e.g. hundreds of millions of rows. In order to use this information in on-demand dashboards, we must be able to retrieve the results of these complex queries in ~100ms. We would also like to build in the flexibility to expand the system over time to handle complex event processing and machine learning tasks. To handle these requirements we are building a system composed of the follow tools:
- Data ingestion using Apache NiFi
- Data warehousing in the Hadoop File System (HDFS)
- Stream processing using Apache Kafka
- OLAP database storage using Druid
- Visualization using Apache Superset
When all integrated, the data flow looks like this:
Below we will walk through what we’ve done so far to build this system and provide instructions that you can follow along to get it set-up yourself for testing. The below is useful to understand the different tools and the processes they rely on.
Note that the below is not designed for a production environment, although the overall structure is the same. In production environments we use a number of automated infrastructure, monitoring, and redundancy tools that are not discussed here.
The below would be much easier to get up and running using Docker or another container system. To get a deeper understanding of how everything works under the hood, we did not use that approach. We would love for someone to contribute a Docker file combining all the below tools, let us know if you do and we will post an update.
Note that the Hortonworks Dataflow (HDF) platform provides a Docker file containing a superset of the above tools, and this GoDataDriven article describes a similar setup and includes a Docker file using Divolte (a clickstream collector) instead of NiFi.
Data Ingestion and Coordination using NiFi
To coordinate the tasks of retrieving data from multiple APIs, minimally transforming it, and appropriately routing it, we use Apache NiFi. We are going to use NiFi to pull historical data from the Ona API and push that data into Kafka and HDFS for later processing. (Future Work has a description of how we do this in production.)
On macOS we can install NiFi with brew install nifi
, and then run it with:
After that NiFi will be available on http://localhost:8080/. Once you have loaded NiFi you can create processor to fetch, store, or process data as well as describe the connections between these processor. To get started quickly here’s a Ona API NiFi flow in XML. This lets you pull data from the Ona API every 60 seconds and route it to Kafka. The imported flow will look like below:
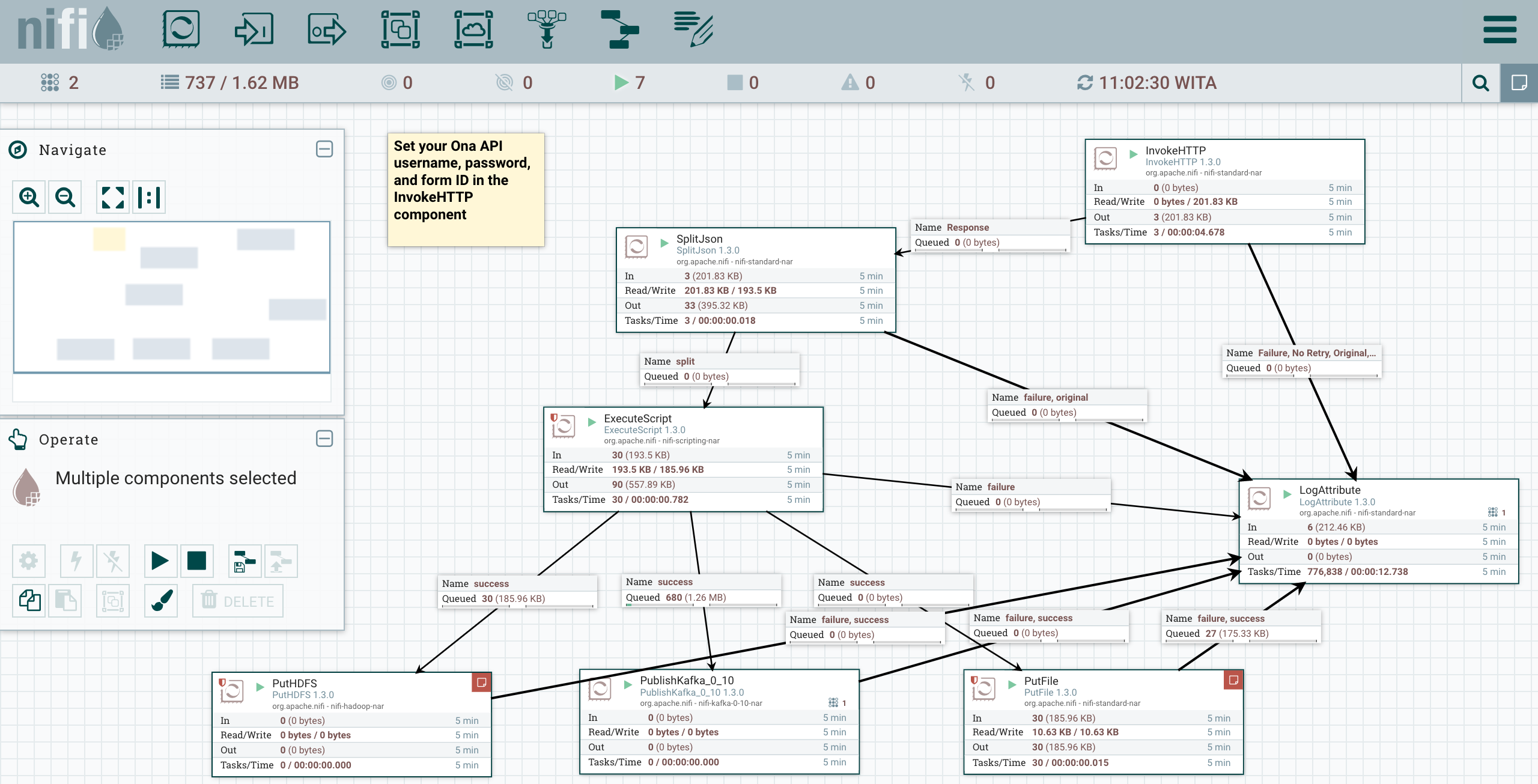
If you read through the flow, you’ll see that we do a number of preprocessing steps before writing the data. When InvokeHTTP
runs it will output API data which is an array of JSON maps. We then convert this batch output into a single output per JSON map using the SplitJSON
component. This will, for example, take a single record that is an array of 10 JSON maps and convert it into 10 records, each of which is a single JSON map.
var flowFile = session.get();
if (flowFile != null) {
var StreamCallback = Java.type("org.apache.nifi.processor.io.StreamCallback");
var IOUtils = Java.type("org.apache.commons.io.IOUtils");
var StandardCharsets = Java.type("java.nio.charset.StandardCharsets");
flowFile = session.write(flowFile,
new StreamCallback(function(inputStream, outputStream) {
var text = IOUtils.toString(inputStream, StandardCharsets.UTF_8);
var obj = JSON.parse(text);
for (var key in obj) {
value = obj[key];
if (typeof value === 'object') {
obj[key] = JSON.stringify(value);
}
obj[key.replace(/\//g, '-')] = obj[key];
delete obj[key];
}
outputStream.write(JSON.stringify(obj, null, '\t').getBytes(StandardCharsets.UTF_8));
}))
flowFile = session.putAttribute(flowFile, 'filename', flowFile.getAttribute('filename').split('.')[0] + '_translated.json');
session.transfer(flowFile, REL_SUCCESS);
}
Because the Ona data is based on the XForm spec, keys in this JSON map may have forward slashes (/
) in them, and values may have nested JSON. If Druid encounters either of these in a JSON map it will not be able to process it. Therefore, we use an ExecuteScript
processor to convert /
s into -
s and nested JSON maps into strings. The ECMAScript Script Body
used by the ExecuteScript
processor is above, this code was inspired by this Fun with Apache NiFi [article]](https://funnifi.blogspot.co.id/2016/03/executescript-json-to-json-revisited.html). (The future Work section below has a description of how we better handle nested JSON in production.)
Because Ona’s data is only accessible securely over HTTPS, we must configure NiFi to work over SSL. To do this we will use the Java keytool
to create a KeyStore and a TrustStore. Then we will import an RSA certificate into our local TrustStore.
To do this, first download a root certificate from the SSL certificate used by Ona (or whatever other HTTPS service your API is hosted behind), here’s Ona’s USERTrustRSACertificationAuthority.crt. Next update the first two lines of the below script with your own passwords and run the script in the same location you’ve downloaded the root certificate:
KEYPASS=changeme
STOREPASS=changeme
echo "Generate server certificate and export it"
${JAVA_HOME}/bin/keytool -genkey -alias server-alias -keyalg RSA -keypass $KEYPASS -storepass $STOREPASS -keystore keystore.jks
${JAVA_HOME}/bin/keytool -export -alias server-alias -storepass $STOREPASS -file server.cer -keystore keystore.jks
echo "Create trust store"
${JAVA_HOME}/bin/keytool -import -v -trustcacerts -alias server-alias -file server.cer -keystore cacerts.jks -keypass $KEYPASS -storepass $STOREPASS
echo "Import CA certificate"
${JAVA_HOME}/bin/keytool -importcert -file USERTrustRSACertificationAuthority.crt -alias RSA -keystore cacerts.jks -storepass $STOREPASS
This will create the KeyStore keystore.jks
and the TrustStore cacerts.jks
with the RSA certificate loaded into it. It will also create a server.cer
, which we can ignore.
To use these new certificates, select the InvokeHTTP
processor, then in its Configuration
under PROPERTIES
, click on the arrow as below:
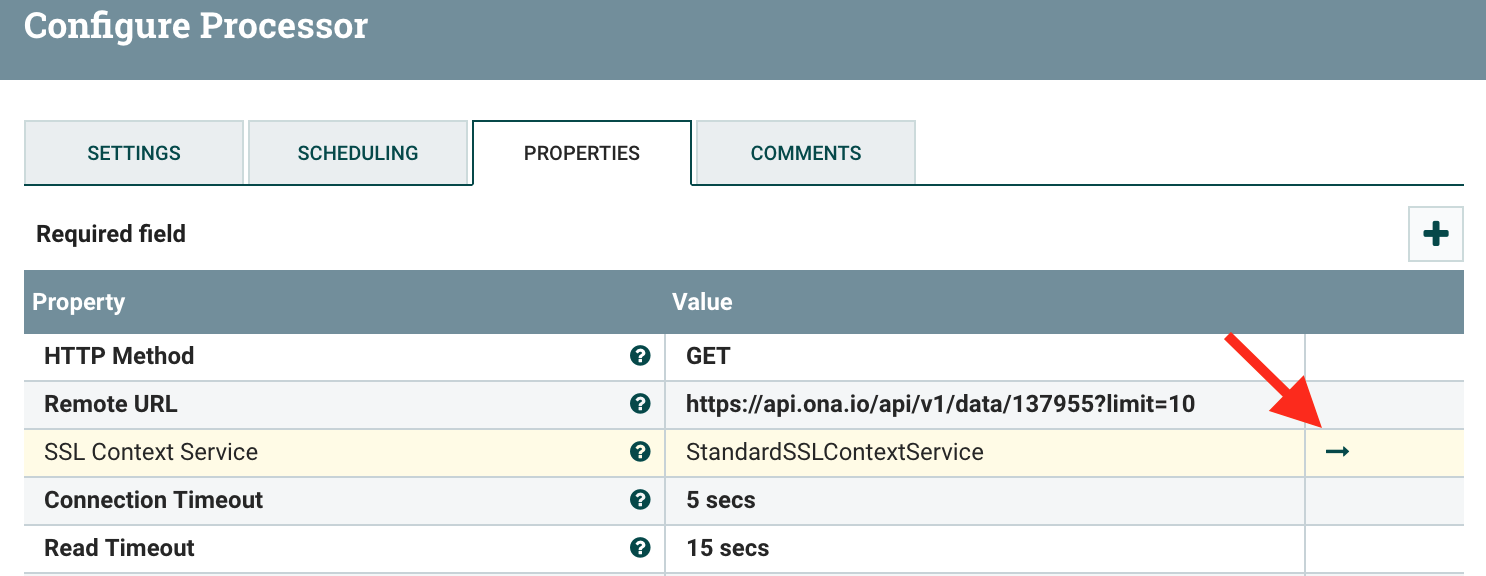
In the new modal window select the StandardSSLContextService
, then hit the info circle to edit its properties. Now set the Keystore Type
and Truststore Type
to JKS, then the Keystore Filename
to the location of your KeyStore and the Truststore Filename
to the location of your TrustStore. Finally, set the passwords to the values you provided in the above script. Hit OK, then use the lightning bolt on the righthand side of the row to enable your new ContextService. If all goes well you should now be able to connect to HTTPS URIs.
To get some data, go back to the flow window, edit the InvokeHTTP
component to set your Ona username, password, and form ID in the URL field. Edit the PutFile
component to specify a directory to write flat files to (this is useful for debugging the data returned by the API and processed by NiFi). Now start the InvokeHTTP
, LogAttribute
, SplitJson
, ExecuteScript
, and PutFile
processes. You should see queues filling and files accumulating in the directory that you have specified. If all looks good, stop the processes and let’s move on to the next step.
Real-time Stream Processing using Kafka
Apache Kafka is a real-time stream processor that uses the publish-subscribe message pattern. We will use Kafka to receive incoming messages and publish them to a specific topic-based queue that Druid will subscribe to. The Druid indexer will read off these messages and insert them into its database. As of this writing, the latest Kafka version that NiFi components support is 0.10, therefore we will install the 0.10 version of Kafka. Use the below commands to download version 0.10, extract it, and change to its directory:
curl -O https://www.apache.org/dyn/closer.cgi?path=/kafka/0.10.0.0/kafka-0.10.0.0-src.tgz
tar xzvf kafka_2.10-0.10.0.0.tgz
cd kafka_2.10-0.10.0.0
For our simple local set-up we will use the default configurations. To manage the Kafka process, and later the Druid processes, we will first start the ZooKeeper task manager:
./bin/zookeeper-server-start.sh config/zookeeper.properties
Leave this running and open a new console in which to start the Kafka process:
./bin/kafka-server-start.sh config/server.properties
To view the clusters and topics we will install the Kafka Manager. This allows us to easily proof check the insertions we are performing, and more quickly surface any errors we might encounter. To install Kafka Manager, first clone the github repository and change to its directory:
git clone https://github.com/yahoo/kafka-manager.git
cd kafka-manager
Next we’ll build the Kafka Manager and run it in the foreground. Since this is a Scala project be sure you have the Scala Build Tool (sbt
) installed, e.g. brew install sbt
.
sbt clean dist
./kafka-manager-1.3.3.13/bin/kafka-manager
With the Kafka Manager running you can visit http://localhost:9000/ to view the current Kafka Clusters, Topics, and Consumers.
Druid is a column-oriented data store, comparable to Vertica, Apache Impala, or Dremel. To power the complex real-time queries that will build the data used in our dashboards we will index data in Druid. Once the data is indexed, we can use Superset for exploratory analysis and to define the JSON queries that we will execute against the Druid API and use to build our dashboards.
To start up Druid, first get the latest Druid source code, decompress it, and change into its directory:
curl -O http://static.druid.io/artifacts/releases/druid-0.10.1-bin.tar.gz
tar xzvf druid-0.10.0-bin.tar.gz
cd druid-0.10.0
To start the Druid services required for our use case, we will run five processes. There will be one process each for:
- a historical data server,
- a server broker,
- a server coordinator,
- a server overlord (the master manager),
- and a server middle manager.
To do this, run each of the below commands in a separate console window:
java `cat conf-quickstart/druid/historical/jvm.config | xargs` -cp "conf-quickstart/druid/_common:conf-quickstart/druid/historical:lib/*" io.druid.cli.Main server historical
java `cat conf-quickstart/druid/broker/jvm.config | xargs` -cp "conf-quickstart/druid/_common:conf-quickstart/druid/broker:lib/*" io.druid.cli.Main server broker
java `cat conf-quickstart/druid/coordinator/jvm.config | xargs` -cp "conf-quickstart/druid/_common:conf-quickstart/druid/coordinator:lib/*" io.druid.cli.Main servercoordinator
java `cat conf-quickstart/druid/overlord/jvm.config | xargs` -cp "conf-quickstart/druid/_common:conf-quickstart/druid/overlord:lib/*" io.druid.cli.Main server overlord
java `cat conf-quickstart/druid/middleManager/jvm.config | xargs` -cp "conf-quickstart/druid/_common:conf-quickstart/druid/middleManager:lib/*" io.druid.cli.Main server middleManager
After starting the above processes, check the Druid Console at http://localhost:8081 to make sure everything looks good.
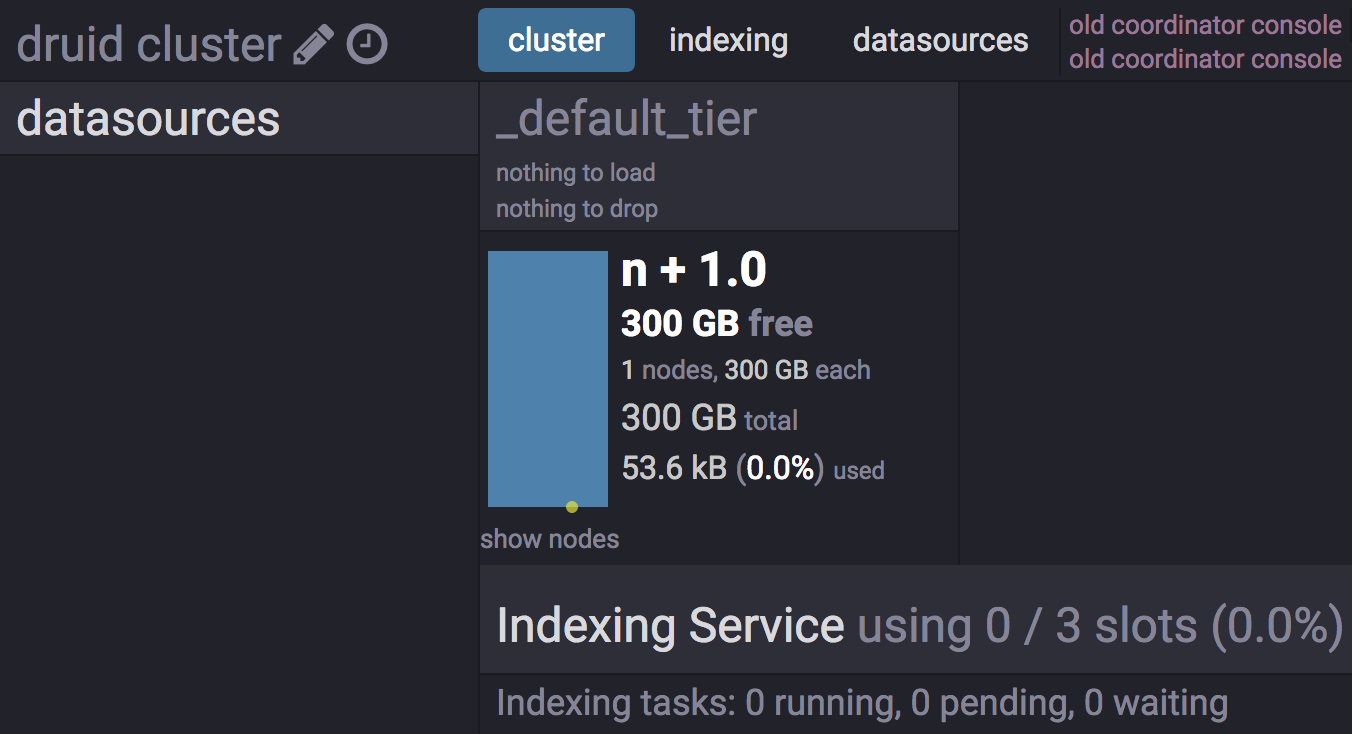
To manage the continuous process that indexes Kafka data, we’ll download, change directories, and run Druid’s Tranquility extension. Assuming you’re in the same directory with the folder that Druid 0.10 was installed, use the following to get the lastest version, decompress it, and then run it:
curl -O http://static.druid.io/tranquility/releases/tranquility-distribution-0.9.0.tgz
tar xzvf tranquility-distribution-0.8.0.tgz
cd tranquility-distribution-0.8.0
bin/tranquility server -configFile ../druid-0.10.0/conf-quickstart/tranquility/server.json
To read from a Kafka stream we will define a configuration file to describe a data source name, a Kafka topic to read from, and some properties of the data that we read. Save the below JSON configuration as ona-kafka.json
and place in the same directory as Druid:
{
"dataSources" : {
"ona-kafka" : {
"spec" : {
"dataSchema" : {
"dataSource" : "ona-kafka",
"parser" : {
"type" : "string",
"parseSpec" : {
"timestampSpec" : {
"column" : "_submission_time",
"format" : "auto"
},
"dimensionsSpec" : {
"dimensions" : [],
"dimensionExclusions" : [
"timestamp",
"value"
]
},
"format" : "json"
}
},
"granularitySpec" : {
"type" : "uniform",
"segmentGranularity" : "six_hour",
"queryGranularity" : "none"
},
"metricsSpec" : []
},
"ioConfig" : {
"type" : "realtime"
},
"tuningConfig" : {
"type" : "realtime",
"maxRowsInMemory" : "100000",
"intermediatePersistPeriod" : "PT10M",
"windowPeriod" : "PT720000M"
}
},
"properties" : {
"task.partitions" : "1",
"task.replicants" : "1",
"topicPattern" : "ona-api-test"
}
}
},
"properties" : {
"zookeeper.connect" : "localhost",
"druid.discovery.curator.path" : "/druid/discovery",
"druid.selectors.indexing.serviceName" : "druid/overlord",
"commit.periodMillis" : "15000",
"consumer.numThreads" : "2",
"kafka.zookeeper.connect" : "localhost",
"kafka.group.id" : "tranquility-kafka"
}
}
This instructs Tranquility to read from the topic ona-api-test
and push the messages that it receives into a Druid data source called ona-kafka
. In the messages it reads Tranquility uses the _submission_time
column (or key) to represent the time stamp. What is particularly unusual about this file is that we set a very large windowPeriod
of PT720000M
. We do this because Druid will ignore any messages with times outside of the windowPeriod
. Because we are using historical data for this test, our time stamps are outside of the typical hour or so window period.
We can start an indexer task using our ona-kafka.json
spec with:
bin/tranquility kafka -configFile ../druid-0.10.0/ona-kafka.json
Alternatively, it is possible to put data directly into Druid and skip the NiFi and Kafka steps. If you would like to do this you can use the below JSON configuration:
{
"type" : "index_hadoop",
"spec" : {
"ioConfig" : {
"type" : "hadoop",
"inputSpec" : {
"type" : "static",
"paths" : "/path/ona-api-demo.json"
}
},
"dataSchema" : {
"dataSource" : "ona-json",
"granularitySpec" : {
"type" : "uniform",
"segmentGranularity" : "day",
"queryGranularity" : "none",
"intervals" : ["2016-07-29/2016-07-30"]
},
"parser" : {
"type" : "hadoopyString",
"parseSpec" : {
"format" : "json",
"dimensionsSpec" : {
"dimensions" : [
]
},
"timestampSpec" : {
"format" : "auto",
"column" : "_submission_time"
}
}
},
"metricsSpec" : []
},
"tuningConfig" : {
"type" : "hadoop",
"partitionsSpec" : {
"type" : "hashed",
"targetPartitionSize" : 5000000
},
"jobProperties" : {}
}
}
}
This assumes you have valid JSON data from the Ona API in the file /path/ona-api-demo.json
that has been transformed to remove /
s in keys and to remove nested JSON in values. It also assumes that the _submission_time
of the data is between the value in intervals
. Go ahead and adjust those based on your demo data.
Assuming you have saved an adjusted configuration file like the above to ona-api-index.json
, you can load a demo JSON file into Druid using:
curl -X 'POST' -H 'Content-Type:application/json' -d @ona-api-index.json localhost:8090/druid/indexer/v1/task
Visualization with Superset
Superset lets us explore the data in our Druid index and build interactive JSON queries which we can later use to power dashboards. To get this up and running we will first need to install Superset. You can follow the instructions in the Superset Getting Started or, assuming you have installed Python’s virtualenvwrapper, use the below commands for macOS, which are mainly taken from there:
# Install required libraries
brew install pkg-config libffi openssl python
# Install the python cryptography library
env LDFLAGS="-L$(brew --prefix openssl)/lib" CFLAGS="-I$(brew --prefix openssl)/include" pip install cryptography==1.7.2
# Create a virtual environment
mkvirtualenv superset
# Upgrade your new virtual environment
pip install --upgrade setuptools pip
# Install superset
pip install superset
# Create an admin user (you will be prompted to set username, first and last name before setting a password)
fabmanager create-admin --app superset
# Initialize the database
superset db upgrade
# Load some data to play with
superset load_examples
# Create default roles and permissions
superset init
Then, start Superset with:
# Start the web server on port 8088, use -p to bind to another port
superset runserver
Finally, we need to add a Druid cluster in SuperSet so that we can access the data stored in the Druid index. To do this, visit the dashboard at http://localhost:8088, then navigate to the Sources
item in the top menu bar then choose the Druid Clusters
option.
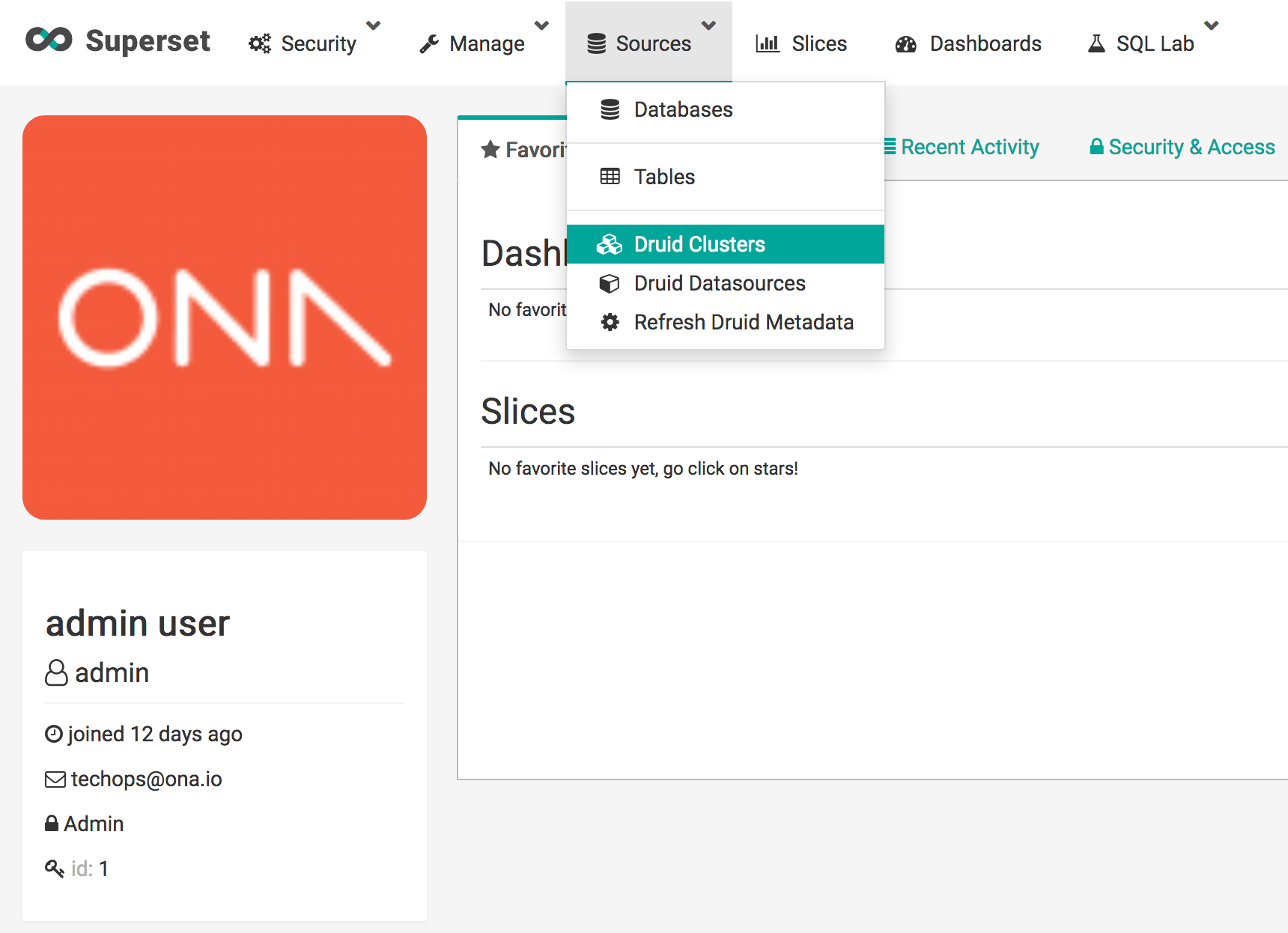
This list should be empty. Hit the teal plus button on the left hand side and enter the below information for your new Druid Cluster.
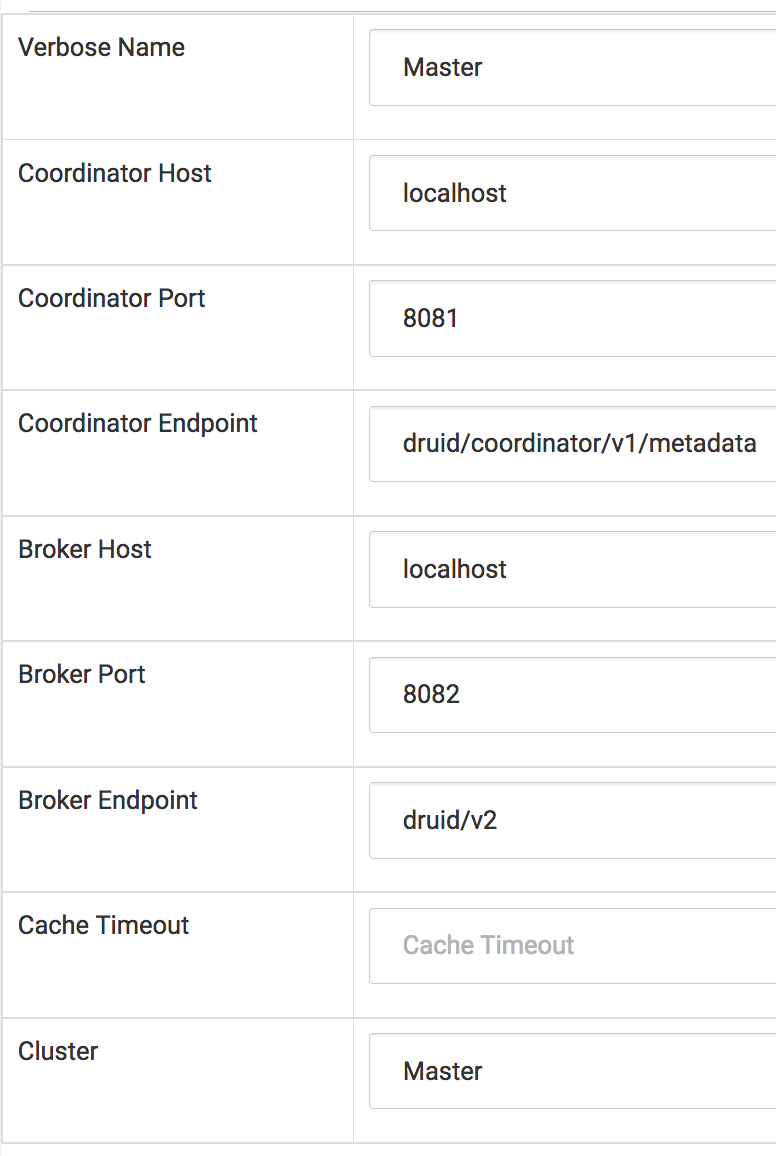
Now return to the Sources
menu at the top and select Refresh Druid Metadata
. After this, again in the Sources
menu, navigate to Druid Datasources
and you will see your ona-json
Datasource. This is the datasource either streaming in, if you used the NiFi and Kafka setup, or from the static JSON file, if you posted data to the indexer.
You can now use SuperSet to explore this dataset. Try creating some queries against it and visualizing the outputs.
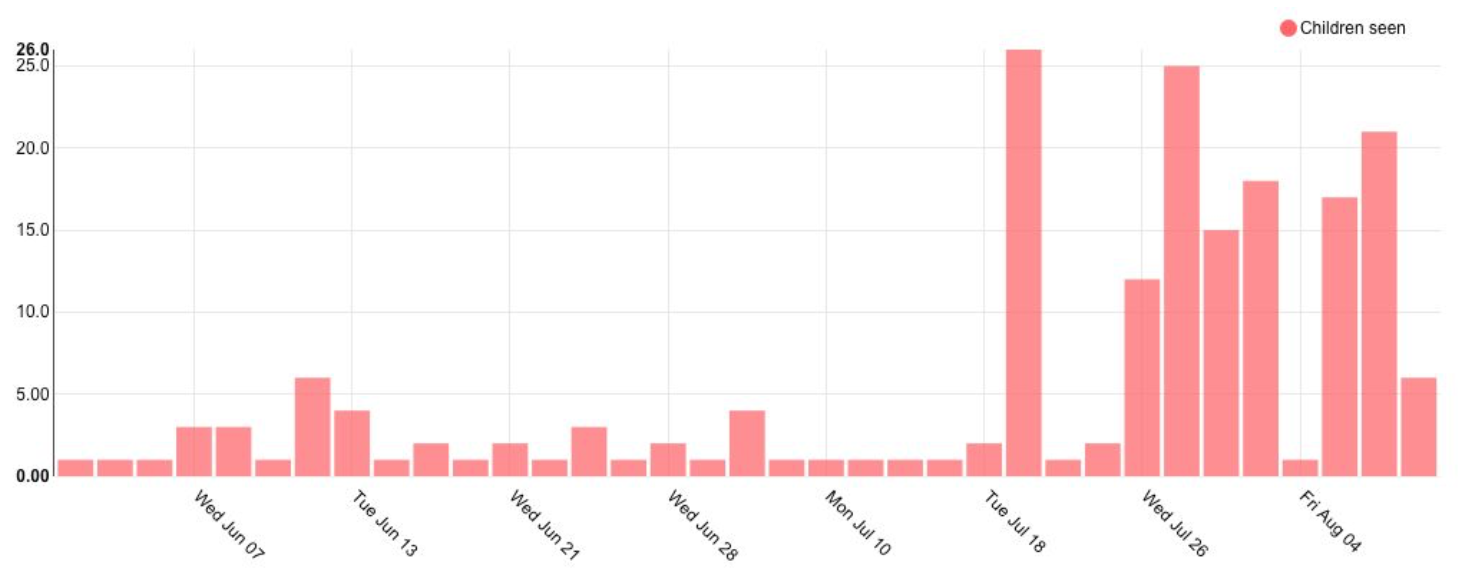
Future Work
Differentiate Batch and Stream Ingestion
Using NiFi to push pulled-data from the API into Kafka was only for the purposes of this proof of concept. In production we will handle streaming data by setting up a listener that receives Ona webhooks containing real-time create, update, and delete events then pushes these into Kafka and HDFS.
We will still use one-time pulls from the API to synchronize historical data from Ona into HDFS and then into Druid. We will begin by using NiFi for this task, but plan to explore alternative tools as well.
Handling Nested JSON
We mentioned above that Druid is not able to index nested JSON, which our records contain, and that we avoid this problem by serializing the JSON values. This is obviously a problem because we cannot run queries against individual key values in the serialized JSON.
One potential solution is to build a semi-relational structure by adding a UUID to the incoming JSON and then inserting that as a foreign parent
key in each nested JSON record with a label
key whose value is the key of the nested JSON in the original record. For example, assuming we reserve the __.+__
namespace pattern for our internal keys:
{
"key1": "value1",
"key2": {
"inner-key1": "inner-value1",
"inner-key2": {
"inner-inner-key1": "inner-inner-value1"
}
}
}
would become three records. The first of which is the outer record and has an assigned __uuid__
:
{
"__uuid__": "AAAA",
"key1": "value1"
}
The second of which is the first nested JSON map referencing its parent through __parent__
and storing its original key in __label__
:
{
"__uuid__": "BBBB",
"__parent__": "AAAA",
"__label__": "key2",
"inner-key1": "inner-value1"
}
The third of which is the doubly nested JSON map only referencing its immediate parent:
{
"__uuid__": "CCCC",
"__parent__": "BBBB",
"__label__": "inner-key2",
"inner-inner-key1": "inner-inner-value1"
}
This structure allows children to easily query parents but not the other way around. To let parents query their children, we could store the child UUID as the value for the what used to be a nested JSON map:
{
"__uuid__": "AAAA",
"key1": "value1",
"key2": "BBBB"
}
The major weakness of this approach is that it adds a significant amount of structural complexity to the datasets. Relatedly, if we expected all our records to have the same key-value pairs, they no longer would. An alternative approach is to flatten our data by pushing all nested keys to the top layer and renaming these keys using the parent keys as prefixes. Again reserving the __.+__
namespace pattern for generated keys and also using it as a separator, this would produce a final JSON map of:
{
"key1": "value1",
"__key2__inner-key1__": "inner-value1",
"__key2__inner-key2__inner-inner-key1__": "inner-inner-value1"
}
when applied to same example dataset.